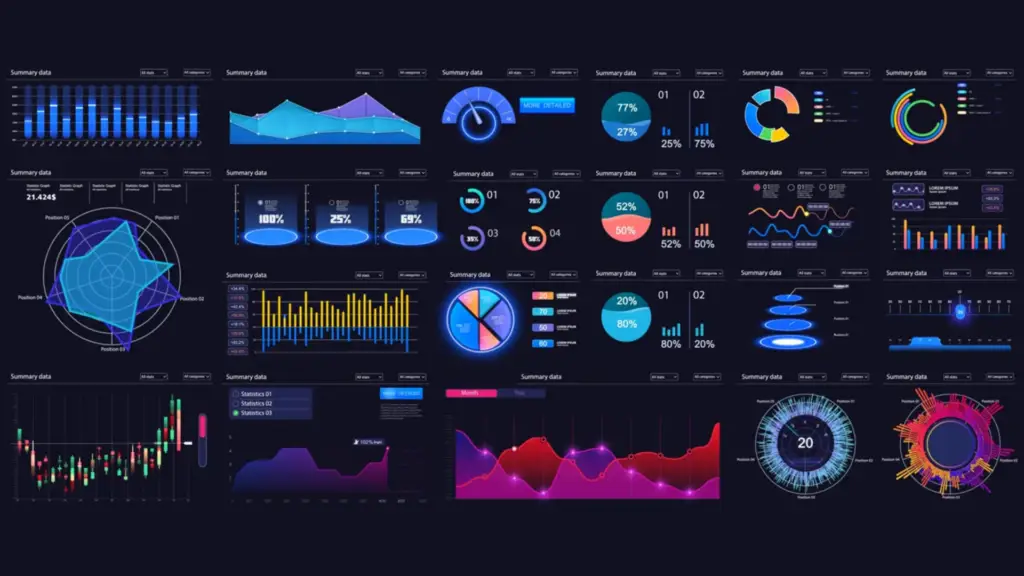
Introduction to Data Visualization
Data visualization is using graphical representations to convey complex information in an easily understandable format. Imagine a traffic light and think:
why red is for stop and green for go and not vice-versa ?
or why do we have signs in sign-boards when there already is text (don’t park, stop etc) ?
There are so many such examples, the underlying concept remains the ease of understanding of visuals – easily and quickly. In this topic, we will explore the significance and benefits of data visualization, understanding the various types of visualizations, and its application in different domains.
Data visualization plays a crucial role in data analysis, enabling analysts and decision-makers to gain valuable insights and trends from datasets. It is widely used in exploratory data analysis to uncover patterns, reporting to present findings and storytelling to communicate data-driven narratives effectively.
Data Visualization Fundamentals
Data visualization is an essential skill for data professionals as it enables them to effectively communicate insights and patterns hidden within datasets. In this topic, we will cover the fundamental concepts of data visualization and explore various chart types with real-world examples.
Data Preparation and Cleaning
Before diving into visualization, it is crucial to ensure that the data is prepared and cleaned appropriately. Some of the data preparation tasks could include :
- handling missing values through imputation, removal, interpolation
- data cleaning
- dealing with outliers
- data formatting to ensure that the data is in the desired format, such as numeric, date or text
Understanding Data and Visualization
In this section, we will delve into the relationship between data and visualization, emphasizing the importance of understanding data types and visual representations. Effective data visualization relies on grasping the nuances of data and employing appropriate visual elements for better communication.
Data Types and Visual Representations
Data comes in various types, such as numerical, categorical, temporal and textual. Each data type requires a specific approach for visualization to ensure that the most suitable visual representation is used.
Quantitative (Numerical) Data: Represents quantities and is measured in numbers. It can be further divided into:
- Discrete Data: Countable data, often represented by whole numbers. Examples include the number of students in a class, the number of cars in a parking lot, etc.
- Continuous Data: Data that can take any value within a range. These are usually measured and include examples such as height, weight, temperature, etc.
Qualitative (Categorical) Data:: describes categories or groups and is not measured numerically. It can be further divided into:
- Nominal Data: Data that represents categories without a specific order. Examples include gender, nationality, types of fruit, etc.
- Ordinal Data: Data that represents categories with a specific order, but the differences between the ranks are not measurable. Examples include rankings (1st, 2nd, 3rd), satisfaction ratings (satisfied, neutral, dissatisfied), etc.
Visual Perception and Design Principles
Effective data visualization relies on understanding how our brains perceive visual information. To create impactful visualizations, consider the following design principles:
- Color Choice: Use colors judiciously to convey information and avoid overwhelming the viewer. Consider color palettes that are accessible and meaningful, enhancing the overall aesthetics. Use color strategically to represent data categories, highlight specific points, or create visual contrasts. Ensure that the color choices are meaningful and support the visualization’s objectives.
- Contrast: Employ contrast to draw attention to important elements within the visualization. Ensure that text and data points stand out against the background.
- Consistency: Maintain consistency in the use of labels, fonts, and styling across all components of the visualization. Consistency enhances the clarity of the message.
- Clarity: Keep the visualization clear and uncluttered. Avoid unnecessary elements that might distract from the main insights.
- Storytelling: Organize the visualization in a way that presents a coherent and compelling narrative. Guide the viewer through the data to communicate insights effectively.
- Labels: Include clear and informative labels for axes, data points, and categories to provide context and understanding to the viewer. Labels aid in interpreting the visualization.
- Annotations: Annotations add valuable context to the visualization. They can explain sudden spikes, important events or other significant observations.
- Interactivity: Consider adding interactivity to the visualization to allow users to explore and interact with the data, providing a more engaging experience.
By understanding the relationship between data and visualization and employing appropriate techniques for data types and design principles, you can create visually compelling and insightful data visualizations that effectively communicate complex information to your audience.